Inferring the function performed by neural circuits
Neural circuits perform a diverse range of functions, from encoding sensory signals to storing memories and controlling muscle movements. A long-standing challenge is to work out which functions are performed by different circuits, and how. For example, an influential theory - 'efficient coding' - posits that low-level sensory neural circuits have evolved so as to transmit maximal information about relevant sensory signals. But what signals are 'relevant' to the organism and what are the constraints faced by a given neural circuit? The answer to these questions are not given by the theory; they must be inferred from data. I work on developing ways to do this.
​
In collaboration with Gasper Tkacik at IST and Olivier Marre at the Vision Institute, I developed a general theory showing how neural codes should vary depending on the goals and constraints of the network (e.g. if the network is optimised to make predictions, or efficiently reconstruct previous stimuli). This work is based on our earlier paper on variational information bottleneck (here's a video of my NeurIPS talk).
​
Recently, I proposed a framework for inferring the function performed by neural networks from data. Here, I showed how a well-established framework (called inverse reinforcement learning) for estimating an agent's goals (such as an animal looking for food or a person driving to work) could be used to infer the function performed by a recorded neural network.

Optimal coding in the retina
I am collaborating with Olivier Marre to test our theories of optimal coding in the retina. The retina provides an ideal test system for these purposes: on the one hand one can record from large populations of neurons while precisely controlling their input; on the other hand, retinal neurons exhibit a large range of diverse and complex behaviours, which we are only beginning to understand. Of particular interest is how different ganglion cell types could be required to perform different functions goals/constraints. I am also interested in understanding how (and why) retinal neurons adapt to the temporal statistics of visual signals.

Strategies for improving visual restoration
A promising strategy to restore vision to blind patients is using retinal prosthetics, which replace the eye's photoreceptor cells by electrically stimulating neurons in the retina. However, currently, the visual acuity that can be achieved with retinal prosthetics is low, limiting their use in many real world tasks. I am interested in how the visual performance achieved with such devices could be improved by optimising how visual signals are transformed into electrical stimulation. I am currently working on a closed-loop experiment, where we will use feed-back from patients' responses in visual tasks to optimise the electrical stimulation that we provide to them. I am first developing this approach on healthy subjects, using computer simulations that mimic the perceptual experience of patients fitted with a prosthetic device. Later, I hope to collaborate with other groups in the Vision Institute to test this approach on real patients.
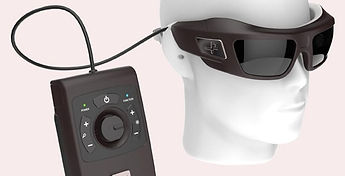